Introduction
At the end of 2013, UnitedHealthcare announced it would drop hundreds of doctors from its network (Kaiser 2013). In Connecticut, UnitedHealthcare terminated about 2,250 physicians, including 810 specialists. In New York City, it terminated 2,100 physicians, affecting some 8,000 patients (data from the Medical Society of New York). UnitedHealthcare justified its decision as necessary to meet rising quality standards and slow the increase in health costs. The controversy over narrow networks predates the Affordable Care Act (ACA), but recently the issue has generated renewed attention as consumers have started to shop for health care coverage through exchanges.
A poll conducted by Kaiser Permanente found that most potential new consumers prefer narrow networks if they come with lower premiums (KFF 2014). Some health plans sold through the ACA exchanges, such as the silver and bronze plans, use narrow networks that exclude physicians and hospitals perceived to be more expensive. A report from McKinsey found that a third of health plans sold in 20 major markets in 2013 restricted provider choices to narrow networks (McKinsey 2013). A year later, in the same markets, the same carriers offered more policies, but 68% of the plans had limited networks, defined as less than 70% of the city’s top 20 hospitals. In markets analyzed by McKinsey, a broader hospital network offered by the same plan in the same metal tier carried a 26% increase in premium when compared with a more limited network. Only 35% of narrow networks include academic medical centers, and participation of an academic medical center triggers an average premium increase of 10% .
Increasingly, health plans, employers, and accreditation agencies are using proprietary criteria of “health care efficiency” as the basis for rating hospitals and physicians. Health care efficiency is used to justify narrow networks that exclude high-price providers, “tiers” with higher cost sharing for patients who use nonpreferred providers, and reference pricing, making patients liable for costs above a benchmark price. However, little agreement exists among payers, employers, consumers, and regulators on how to define and measure health care efficiency.
In Crossing the Quality Chasm, the Institute of Medicine identifies efficiency as one of the 6 domains of high-quality health care (IOM 2001). Nevertheless, measures of health care efficiency reported in the literature and advocated by consultants, health policy experts, accreditation agencies, employers, and payers are not universally accepted. Most importantly, the relation between cost and quality is not established.
In this paper, we analyze the measures of health care efficiency used in research studies for rating hospitals, and the measures used by the health care industry in quality programs, public reporting, and reimbursement models. We also discuss the need to establish a relationship between efficiency measures and quality measures to separate true health care efficiency from cost of care.
Health Care Efficiency and Cost of Care
According to the American Quality Alliance (AQA), cost of care is a measure of the total health care spending, which includes the total use of resources and unit prices for health care services provided to a patient or a population over time (AQA 2009). The AQA defines efficiency of care as the cost of care associated with a specific level of quality of care. Therefore, measurement of efficiency of care should identify the cost of providing high-quality care, which the IOM defines as care that is safe, timely, equitable, effective, and patient-centered (IOM 2001).
Performance Measures of Efficiency and Cost of Care
Measuring Hospital Performance
Most studies of hospital performance do not identify the relationship between quality and cost of care, and only some have used risk adjustment. Most models of hospital efficiency use as inputs both physical resources (labor, equipment, supplies) and costs of the resources utilized to produce health services (McGlynn 2008). The outputs are the amount of health services provided (hospital discharges, number of procedures, number of physician visits). Hospital efficiency is then calculated as ratio of outputs over inputs. The most reported performance measures related to hospital care are severity-adjusted average length of stay, cost per risk-adjusted discharge, and total cost of the severity-adjusted hospital discharge and outpatient visits. Because these measures don’t specify the associated level of quality of care, most are measures of the cost of care rather than the cost of achieving quality care.
A more complex method, stochastic frontier analysis (SFA) (Rosko 2008), compares an individual hospital’s performance with an ideal “frontier” of best performance. However, most of the earlier SFA studies have produced analyses of operational efficiency and don’t address the quality of care. For example, Valdmanis, Rosko, and Mutter found that most efficient hospitals have lower cost per case mix-adjusted admission, fewer full-time equivalents per case mix-adjusted admission, and higher operating margins (Valdmanis 2008).
Recently, though, SFA studies have included measures of true health care efficiency by adjusting for outcomes and risk (Mutter 2008). For example, Deily and McKay demonstrated that a higher risk-adjusted mortality rate in Florida hospitals was associated with higher hospital cost and reduced hospital operational efficiency (McKay 2008). SFA analyses hold the promise of being able to adjust the cost of care for quality indicators, including hospital readmission and access to ambulatory care, and for risk factors such as patient demographics and burden of illness (Rosko 2010).
Measuring Physician Performance
The measures most commonly used to rate the efficiency of physicians’ performance are the relative value units for services provided per physician per month, the number of patient visits per physician per month, and the cost per episode of care. Most of these measures are used by health plans and accreditation agencies and have been developed by private vendors or agencies such as the AQA, the National Committee for Quality Assurance (NCQA), the Leapfrog Group, the Integrated Health Care Organization, and the Employer Health Care Alliance Cooperative (McGlynn 2008).
These proprietary measures are used to develop cost profiles of physicians. They calculate the ratio between the costs of resources used (input) and the amount of episodes of care rendered to individual patients or the total care provided to a specific population over a certain period of time (output).
Most physician cost-profiling methodologies involve assigning episodes of care to individual physicians. An episode of care covers all the care a patient receives during the course of treatment for a specific illness or condition, or for a medical event in a delineated period of time. Each physician’s relative cost is obtained by calculating the ratio of actual (observed) cost of care to the average (expected) cost for similar types of care provided by peer groups.
With the episode-of-care approach, health services are grouped into episodes of care provided to individuals over a set period of time. Efficiency is measured by analyzing the amount of physical and financial resources used to produce an episode of care. Commonly used episode-based measures are episode treatment groups (ETGs) and procedure episode groups (PEGs)(OptumInsight 2014); the medical episodes groups (MEGs) (Truven 2014); and the CCGroup Market-basket System (Cave 2014). All use insurance claims to create groups of episodes of care based on dates of services and related diagnosis codes.
The ETG model identifies all services—including pharmacy—that relate to a patient’s distinct episodes of care. The ETG classification system assigns diagnosis, procedures, and pharmacy codes into 574 groups that serve as benchmarks for comparative analysis. The PEG methodology is the version of the ETG, used to identify surgical procedure episodes and the services related to those episodes. The MEG model applies the disease-staging approach to classify discrete episodes of care into disease stages. The disease-staging criteria define levels of biological severity or pathophysiologic manifestations for specific medical conditions. Contrary to the ETGs, treatments are not part of the disease-staging classification. The CCGroup Market-basket System compares an individual physician’s use of financial resources to a specialty-specific peer group using a standardized set of medical condition episodes adjusting for patient case mix and health status.
The population-based measures analyze the costs or resources used to care for a specific risk-adjusted patient population during a specific period of time. Population-based models are used when the care during that specific period of time can be reliably attributed to a single entity, such as a primary care provider or group practice. They use diagnosis-based, case-mix methodology that evaluates a population’s past or future health care utilization and costs. The population models most commonly utilized by health plans and consultants are Relative Resource Use (RRU) (NCQA 2014), Adjusted Clinical Groups (ACG) (Johns Hopkins 2014), Clinical Risk Groups (CRG) (3M 2014), Diagnostic Cost Groups (DxCG) (Verisk 2014), and the Provider Performance Measurement System (PPMS/Health Dialogue) (Healthdialog 2014). Here is a brief description of the population models:
- RRU evaluates the average resource use for health plan members with a particular condition compared with their risk-adjusted peers. Inputs are the standardized prices and the amount of physical resources used. Outputs include quality measures.
- ACGs are used to determine the morbidity profile of patient populations and to build reimbursement programs based on comparisons of utilization of resources and outcomes across populations.
- CRGs provide a way to consider illness and resource utilization of a full range of patient types, including low income, elderly, commercial beneficiaries, and those with disabilities. 3M CRGs use standard claims data and, when available, additional data (eg, pharmaceutical data, functional health status) collected longitudinally to assign each individual to a single, mutually exclusive risk group. Each 3M CRG can be used to predict health care utilization and costs on a prospective as well as retrospective basis.
- The DxCG model produces clinical groupings from administrative data on the basis of age, sex, and diagnosis. Some DxCG models include drug utilization. Through hierarchies, the model constructs a relative risk score (see box on page 40) that is used to measure the expected resource use based on the patient’s “illness burden.”
- PPMS focuses on the use of health service resources at a given level of comorbidity over a predetermined period of time and unexpected variations in care effectiveness, preference, and supply-sensitive care through the continuum of inpatient and ambulatory settings.
Here are some examples of application of physician performance measures:
Clinical Performance Improvement (CPI), Massachusetts
In 2003, the Group Insurance Commission of Massachusetts established the Clinical Performance Improvement (CPI) Initiative to make quality and cost information available to the public (Alteras 2007). The CPI uses the ETG model. After calculating the total cost of all claims in an episode, episodes are assigned to individual physicians. On the basis of average costs calculated for each specialty, physicians’ cost performance is compared within their specialty using the ratio-of-observed-to-expected costs (O/E ratio) or efficiency index (EI). Ratios above 1.0 indicate relative inefficient performance; those below 1.0 indicate relative efficient performance.
Blue Cross Blue Shield, Special Provider Network, Texas
Blue Cross Blue Shield of Texas has created a special provider network comprising health care providers that met an appropriate risk-adjusted cost index (Lake 2007). Using average cost per episode and adjusting these costs by diagnostic cost group risk scores, the plan compared the costs and quality of their providers and established reimbursement programs based on the risk-adjusted cost index. In this case, the plan’s intent was to reimburse providers on the basis of the risk-adjusted disease burden of the population they treated so physicians with healthier patients would not be overcompensated and those with sicker patients would not be undercompensated.
The UnitedHealth Premium designation program, Aetna’s Aexcel, and Independence Blue Cross’s Integrated Provider Performance Incentive Plan
The UnitedHealth Premium designation program (UnitedHealth 2014) combines quality and cost measures. Physicians must meet first quality designation and then can be considered for cost performance designation (this is referred to as the gated approach). Physicians who meet both quality and cost performance measures receive 2 stars. Those who meet only quality measures receive 1 star. Cost performance is assessed by comparing the percentile rankings of the physician episode costs with a peer group within the same geographic area and specialty. In order to meet the quality criteria, physicians must perform at a level that meets or exceeds the 75th percentile performance for all physicians measured. ETG and PEG software generate episodes of care and allow for case mix and severity adjustments. Inpatient procedures are risk-adjusted by 3M CRGs severity of illness level. Cost performance analysis is based on total cost—a combination of resource utilization, resource mix, and unit cost—for an episode of care. Episodes include all services delivered to a patient, including those of other physicians or clinicians, related to a specific procedure or treatment of a condition. Episodes include dollars paid to the physician for direct services as well as facility costs and ancillary services that the software logic determined were related (eg, medications and diagnostic tests). Complete ETG episodes are attributed to the physician responsible for at least 30% of the total costs. The sets of comparable episodes for all peer group physicians are combined and ranked from lowest to highest percentile. Further analysis is conducted to determine whether the sample size is adequate and whether the difference between physicians’ ranking is statistically significant. UnitedHealthcare disseminates performance information directly to consumers online and via e-mail and print materials.
Aetna’s Aexcel (Aetna 2009) uses similar methodology to award the blue star designations to its specialists in 12 categories. Clinical performance is evaluated on the basis of hospital readmission rates after 30 days; rates of complications during hospital care; and other treatments, by specialty, shown to improve outcomes. The clinical performance measures used by Aetna are endorsed by the AQA, National Quality Forum (NQF), AQA, the American Board of Medical Specialties, the American Osteopathic Association, NCQA, and several specialty societies. Using the same gated approach adopted by United, Aetna evaluates physicians who meet quality standards for “efficiency,” or risk-adjusted optimal use of resources (the cost for services and the number and type of services performed).
The Integrated Provider Performance Incentive Plan (IPPIP) introduced by Independence Blue Cross, Philadelphia, is a hospital/physician rewards program providing a balanced model for high-quality and cost-effective care (George 2011). IPPIP goals are the following:
- Encourage and incentivize enhanced care coordination across the delivery system
- Incorporate measures for improved utilization
- Align primary care, specialist, and hospital incentives
- Complement health care reform-related initiatives, such as ACOs.
Half of the award is based on medical cost management measured via an annual risk-adjusted, per-member, per-month cost target. The other half is based on achieving quality indicators. The quality standards a provider must meet to earn a full reward are as follows:
- 12.5% based on CMS/PHCQA Appropriate Care Measures
- 12.5% based on hospital-acquired infections
- 25% based on Potentially Preventable Readmissions (PPR) rates
Discussion
To address rising health care costs, payers and employers have developed a number of initiatives, including pay-for-performance and value-based tier products, designed to steer patients toward preferred providers. The assumption behind these initiatives is that robust performance measures allow purchasers of care to identify the most efficient providers. Indeed, a number of large employers favor public release of providers’ performance scores and financial incentives to encourage patients to choose providers with high performance scores (Mercer 2007).
Health care efficiency is the cost of care associated with a specific level of quality (AQA 2009). Quality measures are now well established, but measures of health care efficiency are not. A single score that measures the cost of care associated with a specific level of quality doesn’t exist, and there is no rigorous evidence that cost efficiency and high-quality care are proxies for each other. Most studies of hospital performance and most episode groupers used to attribute costs and utilization of resources to individual physicians do not identify the relationship between quality and cost of care. Therefore, current efficiency measures analyze economic performance and provide cost profiling without adjusting for quality.
Aside from health care efficiency, the reliability of conventional cost profiling of individual physicians is questionable. While the validity of different methods of assigning episodes to physicians is established, we cannot be sure they accurately assign the portion of cost variability for which the individual physician is truly responsible.
Adams and colleagues estimated the likelihood of cost performance “misclassification” (Adams 2010). The authors used commercial software to construct episodes of care from claims data provided by 4 health plans in Massachusetts. Overall, 59% of physicians had cost-profile scores with reliabilities <0.70 (suboptimal reliability), and half of internists and two thirds of vascular surgeons were classified inaccurately as lower cost. Reliability varied by specialty, ranging from 0.05 for vascular surgery to 0.79 for gastroenterology and otolaryngology. The authors concluded that current methods of cost performance may produce misleading results. Indeed, conventional methods do not adjust for differences in the types of services provided by physicians within the same specialty (eg, “generalists” vs “interventionists,” colorectal surgeons vs general surgeons) and don’t account for certain physicians’ practice characteristics (eg, solo vs group practice).
Recently, Timbie and colleagues suggested that comparing cost performance of individual providers with the average costs of the entire peer group may be the primary reason for the low reliability demonstrated by conventional cost-profiling methods, particularly when applied to specialties (Timbie 2012). Instead, the authors have proposed the use of propensity score weighting, which adjusts for variables (covariates) such as practice size and types of services rendered. In their study, each physician was compared with a subset of his or her peer group with a similar episode mix instead of the entire specialty group. Then, cost performance calculated with the propensity score weighting was compared against that obtained with conventional groupers tools (entire specialty used as the peer group). The authors concluded that “propensity score weighting resulted in more reliable relative cost estimates than conventional methods for 70 percent of physicians, because weighting the data significantly eliminated statistical errors and unexplained variances.” The improvement was particularly evident for cardiologists, internists, and orthopedic surgeons. Whether these results will be confirmed by subsequent analyses and whether propensity score methodology (D’Agostino 1998) will be widely accepted to improve the reliability of providers’ cost profiling remains to be seen.
It is also worth noting the need for risk adjustment in comparing providers’ efficiency and reviewing some of the challenges associated with risk-adjustment methods. Current SFA methods calculate hospital efficiency by adjusting economic performance for burden of illness and quality of care. However, SFA methods are mostly confined to research. With respect to risk measures used in conjunction with episode groupers, their proprietary ownership has prevented close scrutiny. Several factors limit the accuracy of risk adjustment models, such as inadequate data, patient socioeconomic status, and even patient preferences. In addition, not all severity measures perform best across all conditions. Nevertheless, Iezzoni has shown that the best approach to risk adjustment is to use specific models shown to perform well for the specific outcomes of interest (Iezzoni 2012). No matter how imperfect, risk adjustment must be part of the health care efficiency equation that includes cost and quality because it improves our understanding of what portion of the variability in cost and quality should be attributed to intrinsic patient factors rather than provider performance.
Conclusion
While the IOM considers efficiency as a characteristic of high-quality care, currently a single measure of the cost of care associated with a specific level of quality of care is not commercially available. In addition, cost-of-care profiles have little correlation with quality measures (Rattray 2004). Therefore, at this time, the most practical approach for analyzing health care efficiency is the gated approach (UnitedHealthcare Premium, Aetna’s Aexcel, Independence’s IPPIP), which involves measuring quality and cost of care separately.
Further research is needed for measurement of health care efficiency to advance and become accepted. To be useful, health care efficiency measures need to assess resource use accurately as an input and health outcomes as an output and account for the variability in the costs of producing high-quality care.
References
3M. 3M Clinical risk grouping software. 2014. http://solutions.3m.com/wps/portal/3M/en_US/Health-Information-Systems/HIS/Products-and-Services/Products-List-A-Z/Clinical-Risk-Grouping-Software (link is external). Accessed June 11, 2015.
Adams JL, Mehrotra A, Thomas JW, McGlynn EA. Physician cost profiling—reliability and risk of misclassification. N Engl J Med. 2010;362:1014–1021.
Aetna. Understanding Aexcel. 2009. http://www.aetna.com/docfind/pdf/aexcel_understanding.pdf (link is external). Accessed June 11, 2015.
Alteras T, Silow-Carroll S. Value-driven health care purchasing: case study of the Massachusetts Group Insurance Commission. Commonwealth Fund; 2007. http://www.commonwealthfund.org/usr_doc/1053_Alteras_value-driven_Massachusetts_case_study.pdf (link is external). Accessed June 11, 2015.
AQA (American Quality Alliance). AQA principles of “efficiency” measures. June 2009. http://www.aqaalliance.org/files/PrinciplesofEfficiencyMeasurement.pdf (link is external). Accessed June 11, 2015.
Cave Consulting Group. CCGroup Marketbasket System modules. Accessed June 11, 2015.
D’Agostino RB Jr. Propensity score methods for bias reduction in the comparison of a treatment to a non-randomized control group. Stat Med. 1998;17:2265–2281.
George J. IBC deals to emphasize incentives. Philadelphia Business J. May 6-12, 2011. http://www.ibx.com/pdfs/providers/communications/news/doug_chaet.pdf (link is external). Accessed June 11, 2015.
Health Dialog. http://www.healthdialog.com. Accessed June 15, 2015.
Iezzoni LI, ed. Risk Adjustment for Measuring Health Care Outcomes, 4th ed. Chicago, IL: Health Administration Press; 2012.
IOM (Institute of Medicine). Crossing the Quality Chasm: A New Health System for the 21st Century. Washington, DC: National Academies Press; 2001.
Johns Hopkins. About the ACG system. 2013. http://acg.jhsph.org/index.php/the-acg-system-advantage (link is external). Accessed June 11, 2015.
Kaiser Health News. UnitedHealthcare dropping hundreds of doctors from Medicare Advantage plans. Dec. 1, 2013. http://kaiserhealthnews.org/stories/2013/december/01/medicare-advantage-unitedhealthcare-narrow-networks-doctors.aspx (link is external). Accessed June 11, 2015.
KFF (Kaiser Family Foundation). Kaiser health tracking poll: March 2014. March 26, 2014. http://kff.org/health-reform/poll-finding/kaiser-health-tracking-poll-march-2014 (link is external). Accessed June 11, 2015.
Lake T, Colby M, Peterson S. Health Plans’ Use of Physician Resource Use and Quality Measures. Final Report. Washington, DC: Mathematica Policy Research Inc. Oct. 24, 2007. http://www.mathematica-mpr.com/~/media/publications/PDFs/healthplans.pdf (link is external). Accessed June 11, 2015.
McGlynn EA. Identifying, Categorizing, and Evaluating Health Care Efficiency Measures. AHRQ Publication No. 08-0030. Rockville, MD: Agency for Healthcare Research and Quality. 2008.
McKay NL, Deily ME. Cost inefficiency and hospital health outcomes. Health Econ. 2008;17(7):833–848.
McKinsey & Co. Hospital networks: configurations on the exchanges and their impact on premiums. Dec. 14, 2013. http://healthcare.mckinsey.com/sites/default/files/Hospital_Networks_Configurations_on_the_Exchanges_and_Their_Impact_on_Premiums.pdf. Accessed June 11, 2015.
Mercer National Survey of Employer-Sponsored Health Plans, 2007.
Mutter RL, Rosko MD, Wong HS. Measuring hospital inefficiency: the effects of controlling for quality and patient burden of illness. Health Serv Res. 2008;43:1992–2013.
NCQA (National Committee for Quality Assurance). Using NCQA’s relative resource use measures to get value: efficient, high-quality health care. http://www.ncqa.org/portals/0/Public%20Policy/NCQA%20RRU%20Fact%20Sheet.pdf. Accessed June 11, 2015.
OptumInsight. Episode treatment groups. http://www.optuminsight.com/transparency/etg-links/episode-treatment-groups (link is external). Accessed June 11, 2015.
Rattray MC, Andrianos J, Stam DT. Quality implications of efficiency-based clinician profiling. 2004. http://carevariance.com/images/quality_Implications_Efficiency-Based_Clinician_Profiling.pdf (link is external). Accessed June 11, 2015.
Rosko MD, Mutter RL. Inefficiency differences between critical access hospitals and prospectively paid rural hospitals. J Health Polit Policy Law. 2010;35:95–126.
Rosko MD, Mutter RL. Stochastic frontier analysis of hospital inefficiency: a review of empirical issues and an assessment of robustness. Med Care Res Rev. 2008;65:131–166.
Timbie JW, Mehrotra A, Hussey P, Adams J. Enhancing the validity of physician cost benchmarking: a novel application of propensity scores to derive customized physician peer groups. Presented at: Academy Health Annual Research Meeting; June 26, 2012; Orlando, FL. www.academyhealth.org/files/2012/tuesday/timbie.pdf (link is external). Accessed June 11, 2015.
Truven Health Analytics. Analyze and manage physician care with medical episode grouper. http://truvenhealth.com/Portals/0/assets/HP_11518_0812_MedicalEpisodeGrouper_SS_Web.pdf (link is external). Accessed June 11, 2015.
UnitedHealth Premium Program. 2014. http://www.beasmartpatient.com/find-the-right-care/unitedhealth-premium-program (link is external). Accessed June 11, 2015.
Funding source: None
Conflict disclosure: None disclosed
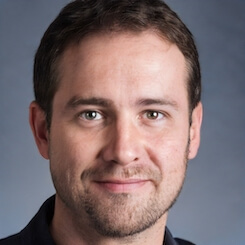
Paul Lendner ist ein praktizierender Experte im Bereich Gesundheit, Medizin und Fitness. Er schreibt bereits seit über 5 Jahren für das Managed Care Mag. Mit seinen Artikeln, die einen einzigartigen Expertenstatus nachweisen, liefert er unseren Lesern nicht nur Mehrwert, sondern auch Hilfestellung bei ihren Problemen.